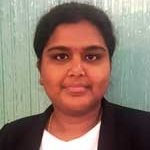
Mobile Network Data carries a lot of advantages in terms of huge sample size that is not possible from the traditional methods, says Madhu Meenakshi Karthikeyan – Managaing Director, Datacorp Traffic Pvt Ltd
India is home to 1.19 billion mobile phone subscribers. Every phone is constantly searching for a mobile cell tower and thereby generating events. These events are sent to the network to inform the location of the mobile phone not only when they are moving but also when stationary. So, the volume of event data that India generates is just enormous. Transport Planners heavily rely on traffic survey data and census data to make informed decisions in relation to policy and investments. Most of the times, this data is based on a small sample size, it is mostly the stated preference and very often will have a lead-time for data collection. On the other hand, Mobile Network Data has extremely large datasets that reveals the preference and has almost no lead-time for data collection.
How a mobile works?
Each mobile phone is connected to one or more cell towers or Base Station Transceivers (BSTs) in that region for transmiting/receiving signals over the radio interface. They have a specific bandwidth and the range varies depending upon the location. These BSTs have a Local Area Code or LAC. A group of BSTs communicate with a Base Station Controller (BSC) and this also controls the communication between the BSTs and the Mobile Switching Centre (MSC), which is the heart of the network. The MSC sets up and maintains calls over the network. In the entire system, only the MSCs are connected to the Public Switched Telephone Network (PSTN).
Event Generation
Events are a log that is generated and stored every time a mobile phone communicates with the BST. The events can be either active or passive. The active events are generated when the phone is active on a call, send/receive text message, when the phone is turned on/off or when moved into a new LAC while on a call. Passive events are generated around every three hours while stationary/unused or when moved into a new LAC. The events are constantly generated either in the form of active or passive events and the location of the phone is recorded all the time.
Privacy Protection
The raw mobile network data is first anonymised to protect the privacy of the users and only the anonymised data in terms of movement is passed on to the next stage. There are also certain criteria for minimum user data in a particular area. If the number falls below the minimum level, the data may not be available considering the privacy.
Mobile Network data contains large volumes of datasets and wide ranges of algorithms are deployed to analyse the data. Mobile Network Data is able to provide insights into the trip purpose (home, work and other) and mode (road, train or air). Figure 1 is a representation of various trips made by an individual in 24 hours based on the events generated from the mobile.
It also represents the origin and destination as per figure 2.
From the contract information that the Network operator holds, it is also possible to understand the gender, demographics and affluence.
Expansion
The data then goes through a process called expansion to ensure the observed trip data which gives a most representative picture of the whole population using a set of algorithms.
Not an alternative
Mobile Network Data has its own limitations and the users of the data must be aware, as the outputs are based on series of inferences from the algorithms and not everything is observed. MND doesn’t come with spatial accuracy and it becomes a challenging task to identify the actual route taken in a busy congested urban situation. MND struggles to split the mode of travel on roads whether the trip was made by car, taxi, bus, HGV, LGV etc. which is an important input in Transport Planning. The trip purpose generated from MND is not very detailed apart from home, work and other. The statistical reliability of algorithms should also be taken into consideration.
Mobile Network Data carries a lot of advantages in terms of huge sample size that is not possible from the traditional methods, it gives a clear picture of the revealed preference, it is collected passively and it has no lead-time for data collection. However, it lacks in identifying the mode of travel, spatial accuracy, trip purpose and the reliability of the expansion process. MND in the current format may not be an alternative for the traditional data collection methods but this is definitely a better alternate data source like other emerging data sources like ANPR, Bluetooth, WiFi, Video Analytics and Machine Learning. Mobile Network Data together with the traditional methods will aid Transport Planners to make informed decisions with their policies and projects.
DataCorp Traffic understands the strengths and limitations of all data sources and use a wide range of Data collection methods like Video Surveys, Automatic Traffic Counters and Classifiers (ATCC) Surveys, Bluetooth, WiFi, ANPR, Video Analytics/ML and Mobile Network Data.